Contents
Our research group participates in consecutive large European projects, founded by the European Union's Horizon research and innovation programme. All these projects have the common goal of internationally mobilising and sharing data and analysis tools in order to mitigate the effects of infectious diseases.
The COMPARE project
COMPARE (Collaborative management platform for detection and analyses of (re-) emerging and foodborne outbreaks in Europe), the predecessor of VEO, running from 2014 to 2019, was a large EU project with the intention to speed up the detection of and response to disease outbreaks among humans and animals worldwide through the use of new genome technology. The aim was to establish a 'one serves all' analytical framework and data exchange platform that allows real-time analysis and interpretation of sequence-based pathogen data in combination with associated data (e.g., clinical, epidemiological data) in an integrated inter-sectorial, inter-disciplinary, international 'One Health' approach.
Our research group has worked in close collaboration with the participating institutions on creating the necessary data sharing and analysis platforms, and we also took part in the analysis and interpretation of the sequencing data obtained from environmental samples collected throughout the project.
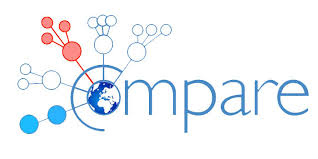
The VEO project
The Versatile Emerging infectious disease Observatory (VEO) project builds upon the foundations laid by COMPARE and aims to further establish a forecasting, nowcasting, and tracking system for the generation and distribution of high-quality actionable information for evidence-based early warning, risk assessment and continuous monitoring of infectious diseases.
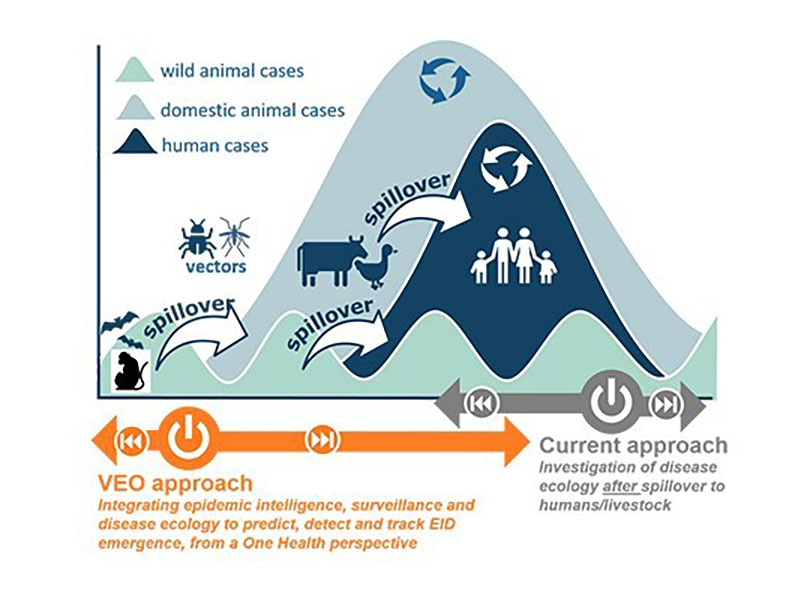
The CSABAI•BIO research group, leading the work package responsible for the development of innovative cloud-based collaborative data mining tools that support data-intensive interdisciplinary collaborations, has created the Kooplex data analysis platform for this purpose. We additionally generate reproducible data analysis pipelines both as demonstrative use cases of the system’s capabilities and as novel scientific research.
The BY-COVID project
The BeYond-COVID (BY-COVID) project was motivated both by the devastating global effects of the COVID-19 pandemic and by the enormous amount of scientific, clinical and epidemiological data made available (in many cases, publicly) online during the last few years. It aims to provide comprehensive open data on SARS-CoV-2 and other infectious diseases across scientific, medical, public health and policy domains. BY-COVID integrates established national and European infrastructures with ELIXIR, BBMRI, ECRIN, PHIRI and CESSDA; and builds on existing efforts, such as the COVID-19 Data Portal and the VEO, maximising efficiency.
It represents an unprecedented and unique interdisciplinary collaboration by bringing together 53 partners from 19 countries and stakeholders from the biomedical field, hospitals, public health, social sciences and humanities.
COVID-19-related work
Due to the recent global spread of the SARS-CoV-2 virus, a significant focus of the above projects has been the management of COVID-19-related datasets and analyses approaches.
Our group has taken an active part in developing the unified analysis pipeline used to detect mutations in raw SARS-CoV-2 sequencing data, the results of which are uploaded to the European COVID-19 Data Portal, containing the analysed datasets of more than 4 million samples. We further designed and continuously maintain the CoVEO PostgreSQL database, in which the above data is stored in a searchable, queriable manner, along with detailed metadata of the relevant samples. Our CoVEO app, developed for the interactive visualisation of the available data in the database, is an integrated part of the COVID-19 Data Portal.
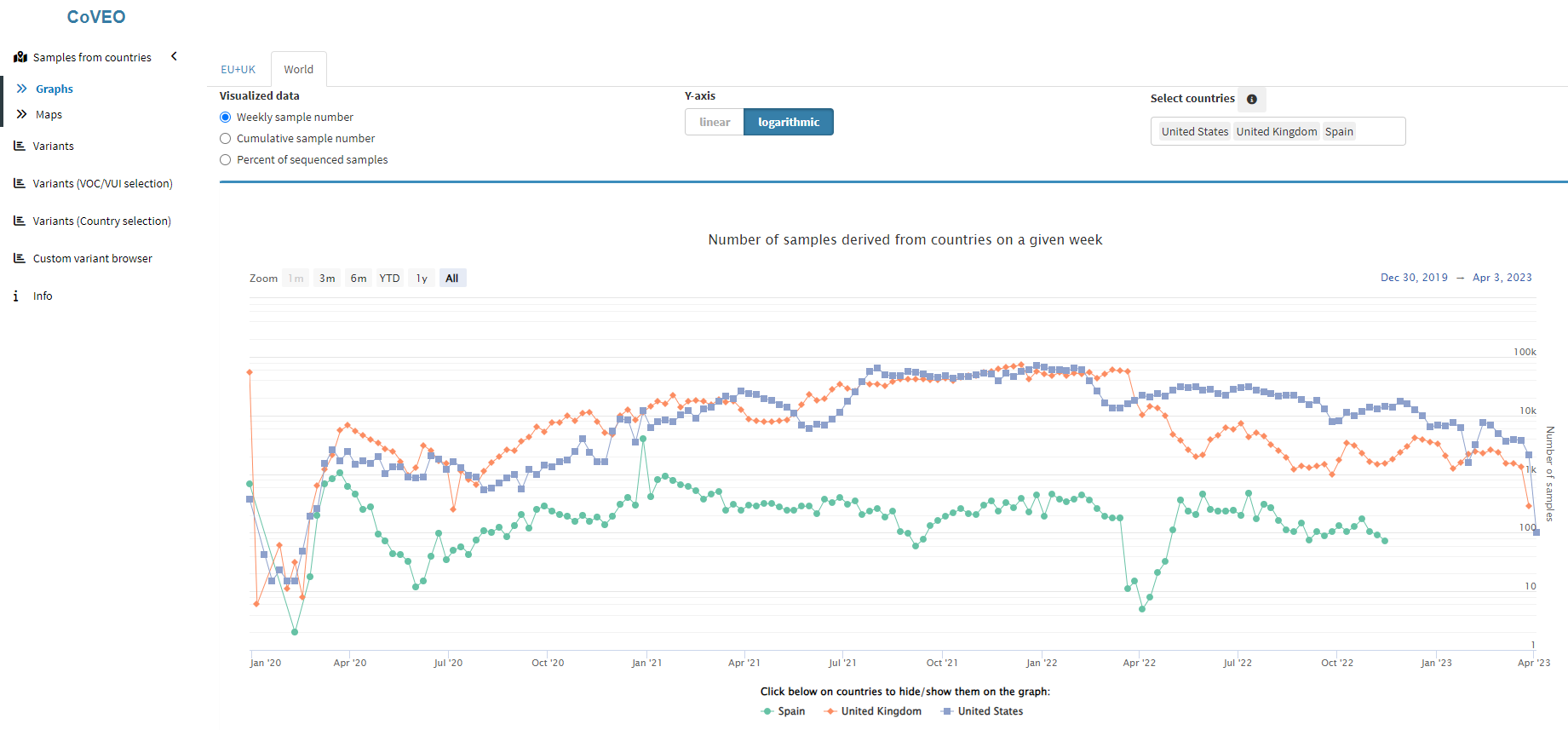
Global sewage surveillance
Human mitochondria in urban sewage
In the Global Sewage Surveillance Project of COMPARE, a global collection of urban sewage was initiated in 2016 to determine the occurrence of antimicrobial resistance genes and infectious disease agents among the healthy human population using metagenomic sequencing. Metagenomic sequencing of urban sewage allows not only the identification of disease-causing agents, like bacteria and viruses, but also a lot of additional information present in the samples, which were not part of the original scope of the study. In the initial analyses, we observed that on average 0.2% of all reads could be assigned to humans.
This relatively small amount of human DNA is insufficient for the detailed profiling of genotype distributions across the populations but limiting the investigations to the mitochondrion (mtDNA) can lead to meaningful results. In our analysis, we reconstructed the local human mtDNA-haplogroup distribution in the cities from where the sewage samples were collected.
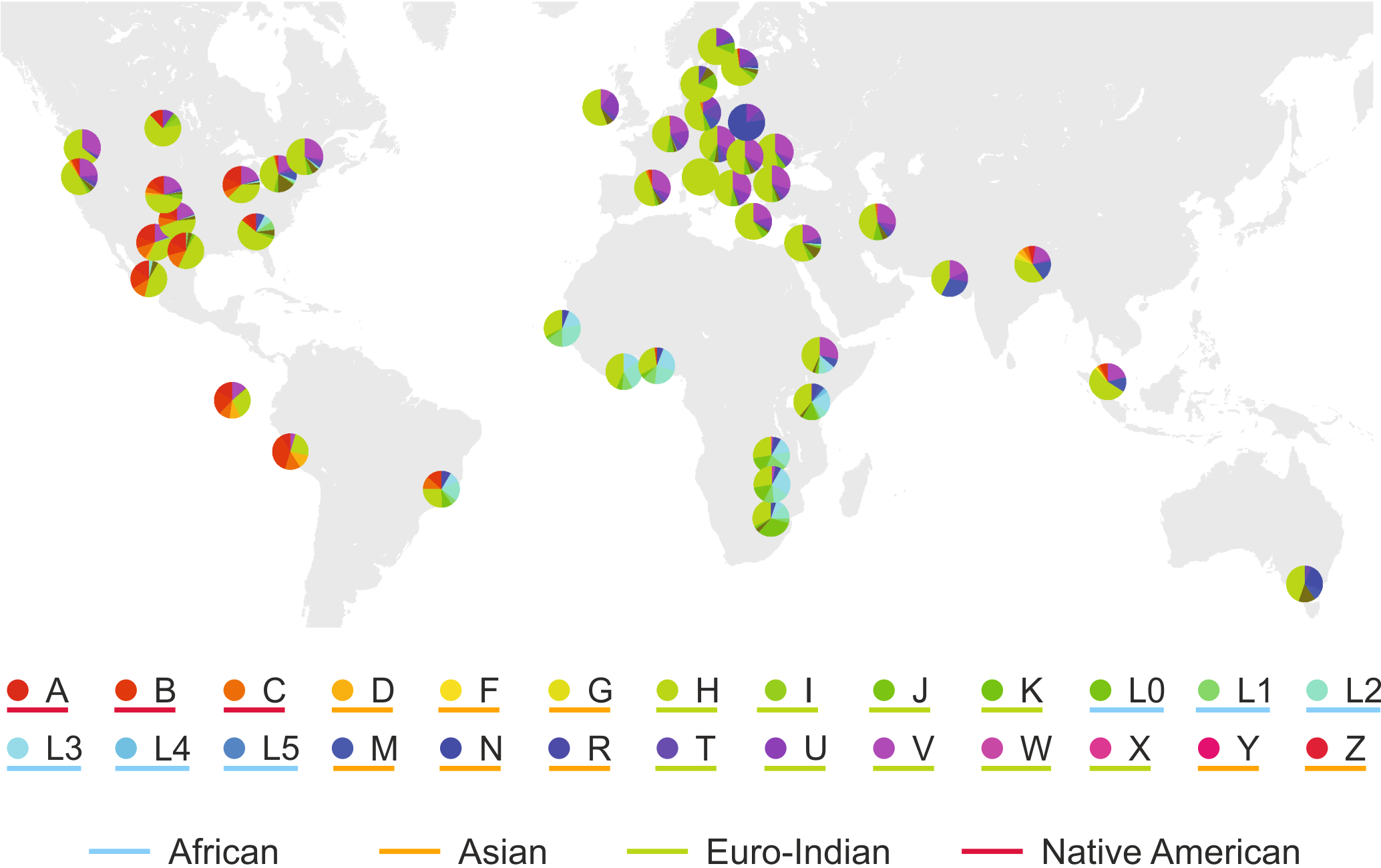
We found that the conclusions based on the analysis of urban sewage show surprisingly great agreement with results previously obtained by careful sampling of specific populations in other studies.
This presents a great possibility for future studies of the ethnic and genetic composition of populations, given that these types of analyses are non-invasive, inherently anonymous, require no informed consent, do not suffer from the limitations of self-reporting and by their nature, provide a well-mixed sampling of the local population. Our results also highlight the future possibility of monitoring demographic effects (such as global migration or the segregation of local communities) in the population in time, as wastewater collection can be accomplished without the need for lengthy preparations and high-cost investments and thus can be repeated as required.
Mosquito tracking
A further promising surveillance approach is relying on smartphones and the Internet to enable novel community-based and digital observatories, where people can upload pictures of disease vectors (e.g. mosquitos) whenever they encounter them. This presents a great advantage over traditional surveillance methods, as these generally rely on catches, which requires regular manual inspection and reporting, and dedicated personnel, making large-scale monitoring difficult and expensive.
An example is the Mosquito Alert citizen science system, which includes a dedicated mobile phone app through which geotagged images are collected. This system provides a viable option for monitoring the spread of various mosquito species across the globe, although it is partly limited by the quality of the citizen scientists' photos. To make the system useful for public health agencies, and to give feedback to the volunteering citizens, the submitted images are inspected and labelled by entomology experts. Although citizen-based data collection can greatly broaden disease-vector monitoring scales, manual inspection of each image is not an easily scalable option in the long run, and the system could be improved through automation. Based on Mosquito Alert's curated database of expert-validated mosquito photos, we trained a deep learning model to find tiger mosquitoes (Aedes albopictus), a species that is responsible for spreading chikungunya, dengue, and Zika among other diseases.
The highly accurate 0.96 area under the receiver operating characteristic curve score promises not only a helpful pre-selector for the expert validation process but also an automated classifier giving quick feedback to the app participants, which may help to keep them motivated.
Related publications
- Mentes et al. Identification of mutations in SARS-CoV-2 PCR primer regions. Sci Rep 12, 18651 (2022). DOI: 10.1038/s41598-022-21953-3
- Kilim et al. SARS-CoV-2 receptor-binding domain deep mutational AlphaFold2 structures. Sci Data 10, 134 (2023). DOI: 10.1038/s41597-023-02035-z
- Rahman et al. Mobilisation and analyses of publicly available SARS-CoV-2 data for pandemic responses. bioRXiv (2023). DOI: 10.1101/2023.04.19.537514
- Pipek et al. Systematic detection of co-infection and intra-host recombination in more than 2 million global SARS-CoV-2 samples. Nat Commun 15, 517 (2024). DOI: 10.1038/s41467-023-43391-z
- Pipek et al. Worldwide human mitochondrial haplogroup distribution from urban sewage. Sci Rep 9, 11624 (2019). DOI: 10.1038/s41598-019-48093-5
- Pataki et al. Deep learning identification for citizen science surveillance of tiger mosquitoes. Sci Rep 11, 4718 (2021). DOI: 10.1038/s41598-021-83657-4
- Amid et al. The COMPARE Data Hubs. Database, 2019, baz136 (2019). DOI: 10.1093/database/baz136